Few things are as misunderstood in drug discovery, and indeed the life sciences more broadly, as the log-normal distribution. (For the subset of us concerned with pharmacology, misunderstanding and misapplication of the free drug hypothesis is tied for first place.) The processes of life, from mitosis to enzymatic catalysis to the endless regulatory feedback loops are all, at their core, multiplicative processes. The very power of life comes from its ability to be a force multiplier. Life would not have taken hold, grown, diversified, or evolved without the power of multiplication.
And yet, when our turn comes to characterize the processes of life with the tools of pharmacology, our human brains seem unable to escape the trap of addition and subtraction. We blindly apply arithmetic tools to a geometric world, and we do so at our peril.
The reasons for this error are, to my eye, several-fold. First, most importantly — and I say this with love — folks working in the life sciences are often short on their math skills. Medicinal chemists are not exempt from this deficit. I often joke that people go into my line of work so that the only number they need calculate ever again is percent yield — and even then we’re really only concerned that the yield is non-zero. Second, to the extent that we have exposure to statistics, life scientists are usually taught only the basics, which are the wrong kind of statistics. In the only probability & statistics class I ever took, the focus was very much on the normal Gaussian distribution and the interrogation of its properties with arithmetic means, standard deviations, confidence intervals, etc. The log-normal distribution was never even discussed. When you put together a natural aversion to the subject matter with the barest of training in the nuances, that’s handing a loaded gun to a toddler and ripe for misapplication.
As a medicinal chemist, I’m a pragmatist. Ours is a business of endless trade-offs and compromises and relentless focus on the end goal of delivering a clinical candidate. So while I built this tweetorial and “went there” to an extent on the math, taking away all of the math details is a secondary objective. If you take nothing else from this tweetorial, take with you:
Nearly all of the numbers you’re relying on in drug discovery should be characterized with geometric (multiplicative), not arithmetic (additive), statistics.
Meaningful differences in biological data are usually at least a half a log (~3-fold) in magnitude. Anything less is probably in the noise.
Ignorance of geometric statistics will lead you astray into making bad decisions.
This is, based on even a cursory inspection of the literature, very much a losing cause. Open up any issue of J. Med. Chem. and within moments you’ll find IC50s and other potency data characterized as arithmetic mean +/- standard deviation, immediately followed by assertions that an IC50 of 100 nM and 150 nM are meaningfully different. For bonus points, this data will be reported to an absurd number of significant figures like 150.6942 nM, but that’s a somewhat different matter.
This behavior is pervasive to the point of normalization in authors, reviewers, and everyone in journal editorial offices. The buck, however, needs to stop with editors. Journals need to have clear standards on proper statistical treatment of biological data, just as they do for proper characterization of new compounds. Bad statistical treatment is easily detected and should be a desk reject, full stop. Only this approach can force the necessary change.
“Well, I don’t know how to do that other thing, and this is good enough for our little study, so it’s not really a big deal” is a common refrain. Ignorance is not only not an excuse in this scenario, it’s downright dangerous — because it leads to flawed conclusions that then propagate forward in the literature. We all own a piece of this issue, so my part in the drama is to call out the bad logic and push for better practices.
Without further ado:

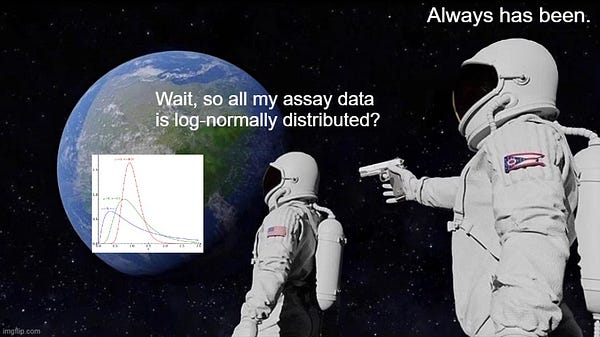

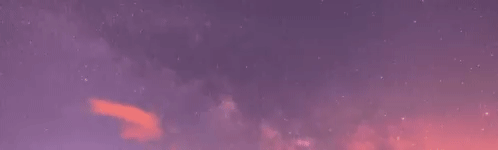

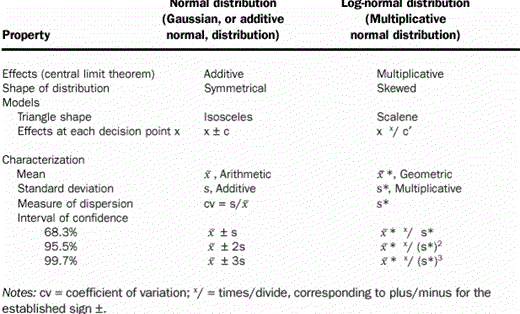

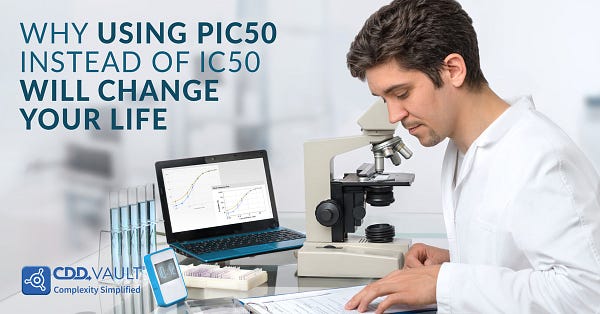

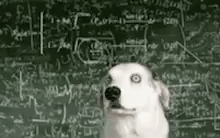

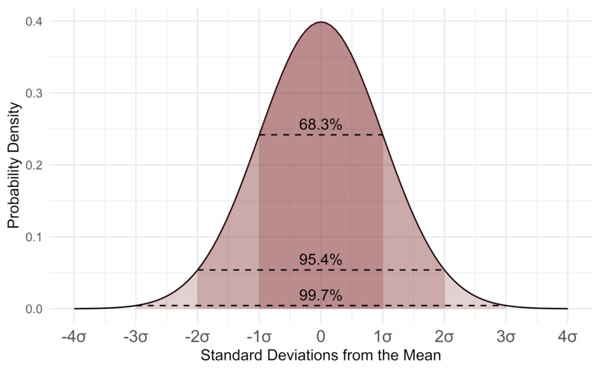

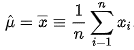
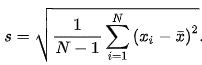

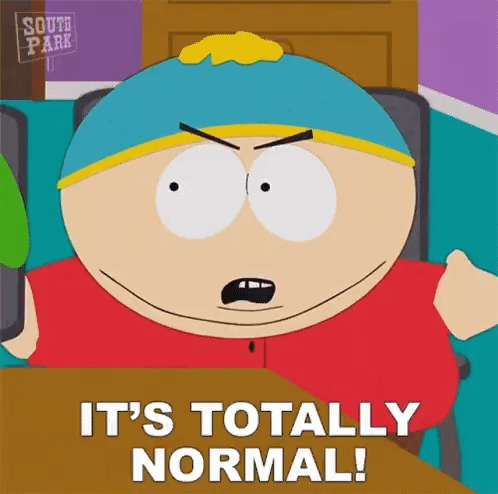

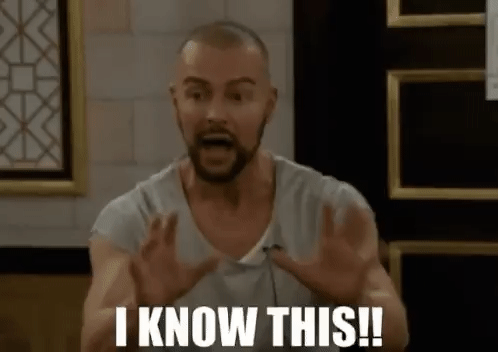

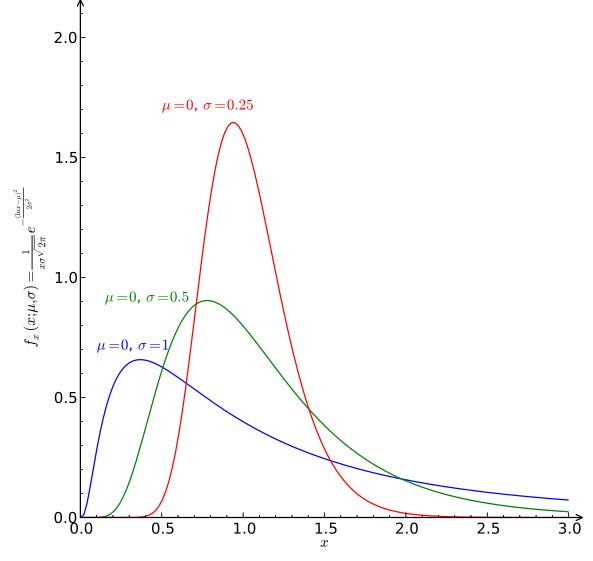

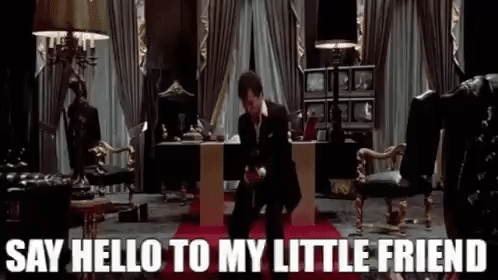

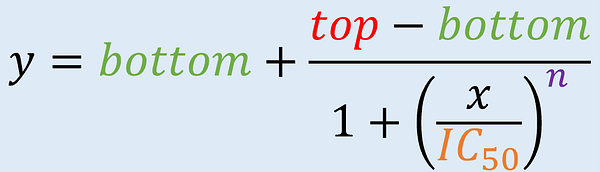

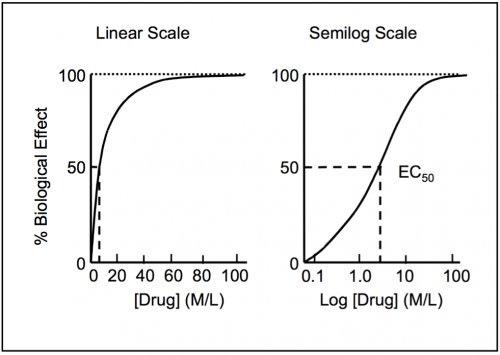

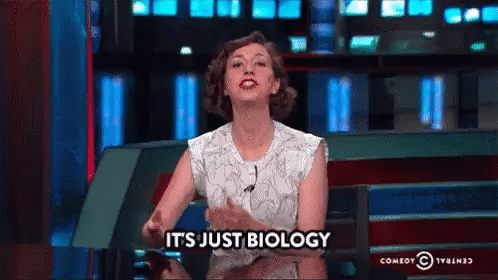

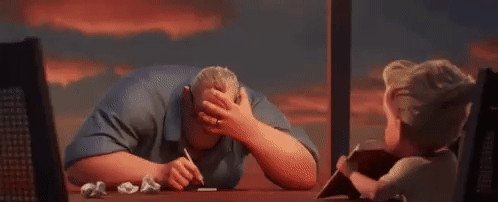


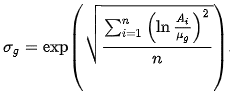

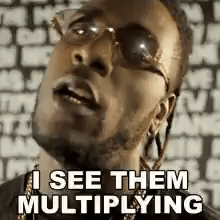



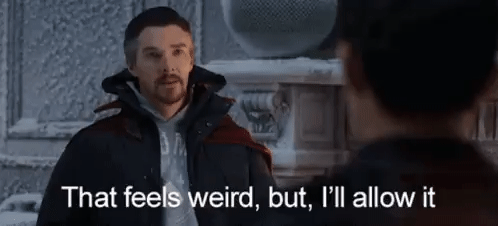

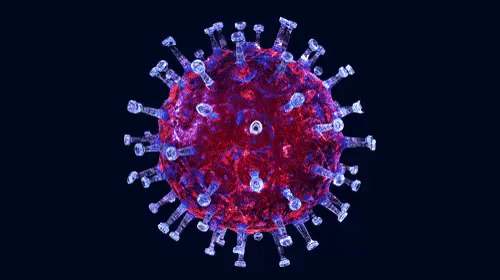

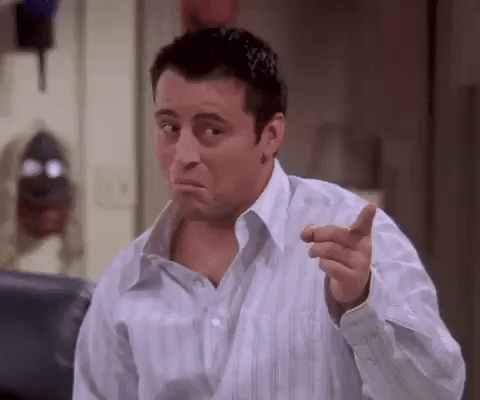



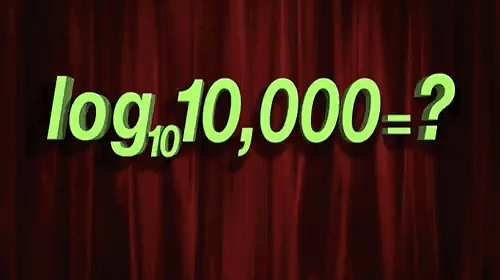

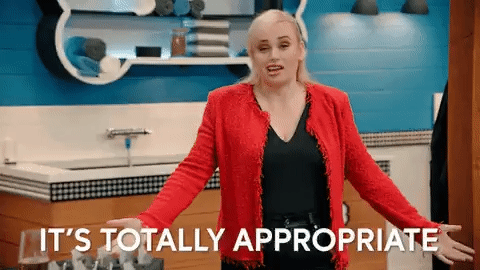

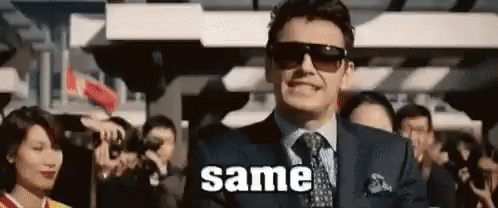

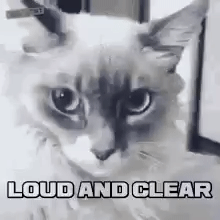

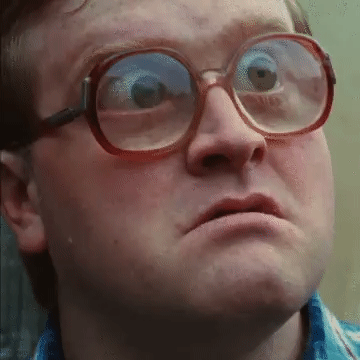

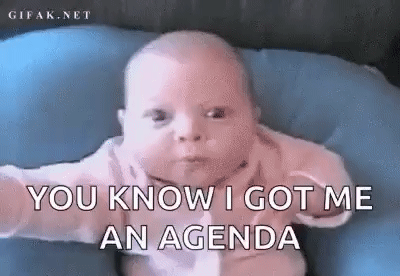

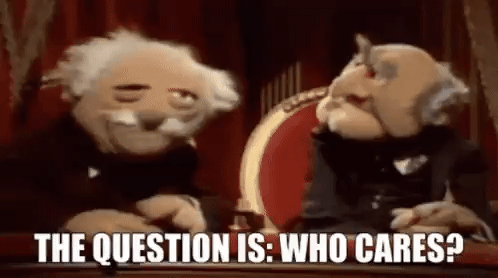